Researchers have achieved impressive results using machine learning to detect dystonias and laryngeal masses that often manifest as vocal abnormalities, with some teams reporting sensitivities as high as 100% (J Voice. 2019;33:947.e11-947.e33). The problem has been replicating those results in real-world clinical practice.
Explore This Issue
November 2020In late September, investigators at Massachusetts Eye and Ear in Boston took a major step in the effort to turn machine learning into a diagnostic tool for the masses. This unique artificial intelligence (AI)-driven diagnostic tool has uncovered, for the first time, microstructural neural networks in the magnetic resonance imaging (MRI) scans of patients with laryngeal dystonia that are proving to be highly reliable biomarkers for the condition (PNAS [Published online September 28, 2020]. doi: 10.1073/pnas.2009165117).
Such a diagnostic tool is sorely needed. Like many vocal abnormalities, laryngeal dystonia (also referred to as spasmodic dysphonia) often is mistaken for other voice disorders. As a result, it can take an average of up to five years after symptom onset for patients to be correctly diagnosed (J Voice. 2015;29:592-594). With the new AI-powered diagnostic tool, in contrast, a definitive diagnosis can be obtained in less than 1 second after scanning a patient’s brain, Kristina Simonyan, MD, PhD, the director of laryngology research at Massachusetts Eye and Ear, told ENTtoday.
In the study, Dr. Simonyan and her colleagues analyzed brain MRIs obtained from 392 patients with three of the most common types of focal dystonia: laryngeal dystonia, cervical dystonia, and blepharospasm. They then compared those MRIs with imaging obtained from 220 healthy individuals and found that the platform diagnosed dystonia with 98.8% accuracy.
Ready for the Clinic
Plans are in place to bring the diagnostic tool out of the lab and into clinical practice. “We designed this platform with exactly that in mind—to quickly get this tool into the hands of practitioners,” Dr. Simonyan said.
To that end, when the team tested the algorithm, they found that in addition to working with a 3.0 Tesla high-resolution MRI, it also was comparably effective when used with a 1.5 Tesla MRI, “a more mainstream scanner available in most clinics around the country, as well as worldwide, so there shouldn’t be a major problem with its widespread use,” Dr. Simonyan said. “One can use conventional MRIs along with the usual types of raw structural images that clinicians order for these patients.”
Given how well the algorithm performed, one might assume that high-powered computers and software are needed to make the platform work. But that isn’t the case, noted co-investigator and first author of the study Davide Valeriani, PhD, a postdoctoral fellow in Dr. Simonyan’s Dystonia and Speech Motor Control Laboratory at Massachusetts Eye and Ear. He explained that the system runs on an easily accessible AI-based deep learning platform called DystoniaNet.
“All you need is a patient’s MRI and an internet connection,” Dr. Valeriani said. “This is a cloud-based platform, and it doesn’t even require installation—it’s truly plug-and-play. Already, we’re getting lots of interest from otolaryngologists who, even as specialists, often struggle to nail down a laryngeal dystonia diagnosis using conventional means.”
Dr. Simonyan said that excitement is understandable, given how few practitioners are trained to detect the subtle and often confusing signs of the voice disorder. “Less than 6% of speech language pathologists actually work in the clinical settings like primary care, where these patients are most likely to be seen,” she explained. “And even if they do make it to an otolaryngologist’s office, there, too, is a knowledge and training gap to overcome: Less than 2% of otolaryngologists are considered to be experts in neurologic laryngeal disorders.”
That neurological basis was the key that led the Mass Eye and Ear researchers to their breakthrough. “Even though laryngeal dystonia manifests as a voice disorder, and it would therefore be reasonable to assume it’s caused at least in part by structural abnormalities affecting the vocal cords, that’s typically not the case,” Dr. Simonyan said. “Rather, it’s caused by a neurological condition that affects speech production. If not for that, these patients’ physical findings appear quite normal in terms of function and anatomy.”
Dr. Valeriani stressed, however, that anatomy isn’t irrelevant when using the new diagnostic platform. “We need the structural brain MRI to rule out other neurological conditions in the current process of diagnosing dystonia,” he said. “But the real power of our system is using structural MRI to make the actual diagnosis, thanks to the machine/deep-learning platform’s ability to see microstructural changes in those MRI scans that simply cannot be seen with the human eye.”
Lest any otolaryngologist reads that quote and worries about being automated out of a job, Dr. Simonyan offered a very large caveat: “This is an objective measure of the disease rather than an AI platform that’s going to replace clinicians, otolaryngologists, or neurologists,” she said. “Their expertise cannot be replaced, but their clinical knowledge can be augmented by this tool—and frankly it needs to be, based on the delayed time to definitive diagnosis and treatment that’s held true for so long.”
NIH Funding Power
The National Institutes of Health (NIH) sees the value of machine learning in otolaryngology, as evidenced by a grant it recently bestowed on Andrés Bur, MD, an assistant professor and director of robotics and minimally invasive head and neck surgery at The University of Kansas (KU) Medical Center in Kansas City. The focus of the grant is to support the use of machine learning to help detect and classify structural laryngeal lesions based on visual images obtained during endoscopy.
On first glance, Dr. Bur noted, the grant work isn’t specifically focused on speech pathology. “But it’s still very related,” he noted. “Laryngeal lesions are often detected because of dysphonia or some other change in the voice. Patients who live in rural communities, which is a big issue in this part of the country, may not have ready access to an otolaryngologist to identify and better characterize the laryngeal lesion and its related voice disorders. That’s where machine learning comes in. The long-term goal of our work is to increase laryngology care access and improve early detection of laryngeal cancers using neural networks.”
We’re very hopeful our approach will become that elusive, accurate vocal test with an actionable snapshot of the acoustic signature that can be followed very accurately over time, much like an EKG or a pulmonary function test. —Tanya Meyer, MD
The link between lesions and voice is partly why Dr. Bur is collaborating with Shannon Kraft, MD, an associate professor in the KU department of otolaryngology–head and neck surgery who specializes in voice disorders, and Guanghui Wang, PhD, an expert in computer vision and assistant professor of electrical engineering and computer science at KU.
Dr. Bur said his team is employing a convolutional neural network to process large sets of images of the larynx obtained from patients treated at his institution. “We’re basically trying to ‘teach’ the network to process the images and determine whether there’s a lesion present and, if there is a lesion, to classify it. And then, based on the results, ideally, we’d like to use this tool to recommend the next diagnostic and/or treatment steps that may be needed.
“At the end of the day, my primary focus is to care for patients with laryngeal cancers,” Dr. Bur continued. “And, unfortunately, I too often see these lesions not being diagnosed promptly. In the case of cancer, that can have catastrophic consequences. We’re using a visual-based approach with machine learning to analyze large databases of images of the larynx obtained from in-office exams to see if we can develop algorithms to facilitate early diagnosis.”
Enlisting Smartphones
Anthony Law, MD, PhD, an expert in machine learning at the University of Washington (UW) in Seattle, is part of a separate research team that already has succeeded in bringing the technology out of the lab and into the clinic, albeit with work needed to warrant more widespread use.
“Our focus has been on using machine learning to diagnose a laryngeal mass,” said Dr. Law, who recently completed his laryngology fellowship at UW and is now on the faculty at Emory University, in Atlanta. “But it’s important to note that, oftentimes, vocal changes are the first sign of an abnormal laryngeal growth, whether malignant or benign. It’s all intertwined.”
Clinician, otolaryngologist, and neurologist expertise cannot be replaced, but their clinical knowledge can be augmented by this tool—and frankly it needs to be, based on the delayed time to definitive diagnosis and treatment that’s held true for so long. —Kristina Simonyan, MD, PhD
Dr. Law’s approach, detailed in an ongoing study with Grace Wandell, MD, an R3 resident in otolaryngology, and Tanya Meyer, MD, a surgeon at UW Medicine’s Head and Neck Surgery Center, was to build a machine-learning algorithm that could be given to primary care physicians to use with smartphones. The system has the capability to analyze whether a patient’s vocal phonatory signal (as recorded on the smartphone) puts them in a high- or low-risk category for a vocal fold mass—and thus for laryngeal cancer—with the need for expedited specialty follow-up care.
Other colleagues on the UW project include Mark Whipple, MD, an associate professor and a bioinformatics researcher at UW Medicine, and Albert Merati, MD, a UW professor with research interests in the diagnostic testing and treatment of vocal fold paralysis.
“The results are preliminary, but we’ve made some real progress that suggests this could be an amazing tool for identifying an individual with a suspicious laryngeal mass sooner, particularly in primary care settings,” Dr. Law said. “We’ve seen in our own practice that there’s often a huge delay in care from the time a patient is seen by a primary care physician to eventually being diagnosed with a mass and evaluated by an otolaryngologist.”
Dr. Law acknowledged that some of the problems that have challenged other machine-learning projects cropped up with this one in practice, including a fall-off in sensitivity. “In the lab, we were great—our accuracy rates approached 90%,” he said. “But when we placed it in Dr. Meyer’s clinic [using smartphone-based telemedicine], it just didn’t generalize as well. We went from audio in the lab that was collected retrospectively on a strobe machine, with all of the usual artifacts in there, to data that were collected on an iPhone. I think something was just lost in the translation.”
Part of the challenge, Dr. Law added, was the dataset. “Like most machine-learning researchers, we’ve had difficulty finding a large enough one to match well to all patients. Still, it’s probably one of the cleanest datasets out there; it’s been independently reviewed by multiple specialists in speech pathology and laryngology. So, we’re confident this will eventually work in practice.”
A Tool Made for Pandemics
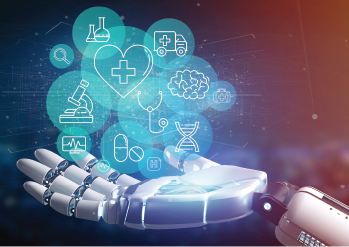
© Production Perig / shutterstock.com
This kind of diagnostic support is critical, particularly during pandemics such as COVID-19, noted Dr. Meyer, who’s also an associate professor of otolaryngology at UW.
“The pandemic wasn’t even a consideration when we started this process,” she said. “Before it hit, performing endoscopy to detect laryngeal abnormalities was a daily in-office procedure, just like listening to someone’s heart. But endoscopy has become a procedure that could be fraught with significant danger to the physician and the caregiving team. [That makes] the ability to actually acoustically follow, not the visual signal of the voice but the acoustic signal of the voice via telemedicine, very powerful.”
Refinements are still needed, Dr. Meyer acknowledged, but machine learning is far superior to previous methods for analyzing vocal disorders. “We have tons of acoustic and vocal tests, but none of them are very useful,” she said. “Whether it’s jitter, shimmer, or any other myriad artifacts we struggle with, none of it has panned out despite their continued use in the clinic [J Voice. 2017;31:382.e15-382.e26]. We’re very hopeful our approach will become that elusive, accurate vocal test with an actionable snapshot of the acoustic signature that can be followed very accurately over time, much like an EKG or a pulmonary function test. This kind of telemedicine-based approach will become critical during the continuing pandemic, where we just don’t have the same access to patients we’ve had in the past.”
Looking ahead, Dr. Bur said that machine learning has the potential to be a truly transformative technology in otolaryngology. But that trajectory depends in part on datasets. “The more data that you have, the more powerful these tools become,” he said. “If you think about an individual clinician who may treat a few thousand patients throughout their career, that’s one thing. But with machine learning, you can incorporate data from millions of patients in an algorithm. That opens up a whole new level of potential discernment, speed, and accuracy.”
Nikki Kean and David Bronstein are freelance medical writers based in New Jersey.
Parkinson’s Disease and Machine Learning
Outside of cancer, some promising work with machine learning in otolaryngology is being done in diagnosing dysphonia and other vocal abnormalities secondary to neurological conditions such as Parkinson’s disease (PD), said Andrés Bur, MD, an assistant professor and director of robotics and minimally invasive head and neck surgery at The University of Kansas (KU) Medical Center in Kansas City. “Researchers have made great progress using machine learning to analyze audio recordings of the voice,” he said. “It’s a natural fit, because the voice changes in PD are very characteristic. And while they might be somewhat easily identified by specialists, the primary care physician might not be so adept. Giving the non-specialist a tool to screen for voice changes that might signal PD is a real advance.”
He cited, as one example, a study by Xu and colleagues, which noted that more than 90% of PD patients suffer from vocal cord disorders. The researchers used a deep neural network recognition and classification method to distinguish the vocal characteristics of patients with PD from healthy controls. Using the technique, they were able to confirm a PD diagnosis in 89.5% of cases, concluding that such technologies “can provide a solid solution for the quick auxiliary diagnosis of PD in [the] early stage.” —DB